-
László Gaál – Google Veo2 tests
https://www.linkedin.com/posts/laszloga_veo2-activity-7278344748464029696-z18_
https://www.linkedin.com/posts/laszloga_veo2-veo2-activity-7279424228779507712-zDgC
https://www.linkedin.com/posts/laszloga_veo2-activity-7280530104722583552-tGgJ
https://www.linkedin.com/posts/laszloga_veo2-activity-7280881794663510016-e8i8
https://www.linkedin.com/posts/laszloga_veo2-activity-7277947758932606976–7i9
https://www.linkedin.com/posts/laszloga_veo2-activity-7283050136446935041-EJGs
-
Bloomberg – Sam Altman on ChatGPT’s First Two Years, Elon Musk and AI Under Trump
https://www.bloomberg.com/features/2025-sam-altman-interview
One of the strengths of that original OpenAI group was recruiting. Somehow you managed to corner the market on a ton of the top AI research talent, often with much less money to offer than your competitors. What was the pitch?
The pitch was just come build AGI. And the reason it worked—I cannot overstate how heretical it was at the time to say we’re gonna build AGI. So you filter out 99% of the world, and you only get the really talented, original thinkers. And that’s really powerful. If you’re doing the same thing everybody else is doing, if you’re building, like, the 10,000th photo-sharing app? Really hard to recruit talent.
OpenAI senior executives at the company’s headquarters in San Francisco on March 13, 2023, from left: Sam Altman, chief executive officer; Mira Murati, chief technology officer; Greg Brockman, president; and Ilya Sutskever, chief scientist. Photographer: Jim Wilson/The New York Times
-
LG 45GX990A – The world’s first bendable gaming monitor
The monitor resembles a typical thin flat screen when in its home position, but it can flex its 45-inch body to 900R curvature in the blink of an eye.
-
DMesh++ – An Efficient Differentiable Mesh for Complex Shapes
https://sonsang.github.io/dmesh2-project
An efficient differentiable mesh-based method that can effectively handle complex 2D and 3D shapes. For instance, it can be used for reconstructing complex shapes from point clouds and multi-view images.
-
Nvidia unveils $3,000 desktop AI computer for home LLM researchers
https://arstechnica.com/ai/2025/01/nvidias-first-desktop-pc-can-run-local-ai-models-for-3000
https://www.nvidia.com/en-us/project-digits
Some smaller open-weights AI language models (such as Llama 3.1 70B, with 70 billion parameters) and various AI image-synthesis models like Flux.1 dev (12 billion parameters) could probably run comfortably on Project DIGITS, but larger open models like Llama 3.1 405B, with 405 billion parameters, may not. Given the recent explosion of smaller AI models, a creative developer could likely run quite a few interesting models on the unit.
DIGITS’ 128GB of unified RAM is notable because a high-power consumer GPU like the RTX 4090 has only 24GB of VRAM. Memory serves as a hard limit on AI model parameter size, and more memory makes room for running larger local AI models. -
Gaussian Splatting OFX plugin for Nuke
https://radiancefields.com/gaussian-splatting-in-nuke
https://aescripts.com/gaussian-splatting-for-nuke
Features
- Import .ply files in Nuke.
- Support Compressed .ply files from SuperSplat
- Crop with Spherical or Box shape.
- Crop with Y Plane.
- Combine up to 10 models in the scene.
- Colorize with Ramp using Spherical or Box shape.
- Reveal model with Opacity Ramp.
- Animate Splat Scale with Spherical or Box shape.
- Each model can be distorted with Noise.
- Render Depth Pass for 3D compose.
- Color correction for each model.
- Real-time with GPU
- Export scene
-
ComfyUI + InstaID SDXL – Face and body swap tutorials
https://github.com/cubiq/ComfyUI_InstantID
https://github.com/cubiq/ComfyUI_InstantID/tree/main/examples
https://github.com/deepinsight/insightface
Unofficial version https://github.com/ZHO-ZHO-ZHO/ComfyUI-InstantID
Installation details under the post
(more…) -
ComfyUI Tutorial Series Ep 25 – LTX Video – Fast AI Video Generator Model
https://comfyanonymous.github.io/ComfyUI_examples/ltxv
LTX-Video 2B v0.9.1 Checkpoint model
https://huggingface.co/Lightricks/LTX-Video/tree/main
More details under the post
(more…) -
The AI-Copyright Trap document by Carys Craig
https://papers.ssrn.com/sol3/papers.cfm?abstract_id=4905118
“There are many good reasons to be concerned about the rise of generative AI(…). Unfortunately, there are also many good reasons to be concerned about copyright’s growing prevalence in the policy discourse around AI’s regulation. Insisting that copyright protects an exclusive right to use materials for text and data mining practices (whether for informational analysis or machine learning to train generative AI models) is likely to do more harm than good. As many others have explained, imposing copyright constraints will certainly limit competition in the AI industry, creating cost-prohibitive barriers to quality data and ensuring that only the most powerful players have the means to build the best AI tools (provoking all of the usual monopoly concerns that accompany this kind of market reality but arguably on a greater scale than ever before). It will not, however, prevent the continued development and widespread use of generative AI.”
…
“(…) As Michal Shur-Ofry has explained, the technical traits of generative AI already mean that its outputs will tend towards the dominant, likely reflecting ‘a relatively narrow, mainstream view, prioritizing the popular and conventional over diverse contents and narratives.’ Perhaps, then, if the political goal is to push for equality, participation, and representation in the AI age, critics’ demands should focus not on exclusivity but inclusivity. If we want to encourage the development of ethical and responsible AI, maybe we should be asking what kind of material and training data must be included in the inputs and outputs of AI to advance that goal. Certainly, relying on copyright and the market to dictate what is in and what is out is unlikely to advance a public interest or equality-oriented agenda.”
…
“If copyright is not the solution, however, it might reasonably be asked: what is? The first step to answering that question—to producing a purposively sound prescription and evidence-based prognosis, is to correctly diagnose the problem. If, as I have argued, the problem is not that AI models are being trained on copyright works without their owners’ consent, then requiring copyright owners’ consent and/or compensation for the use of their work in AI-training datasets is not the appropriate solution. (…)If the only real copyright problem is that the outputs of generative AI may be substantially similar to specific human-authored and copyright-protected works, then copyright law as we know it already provides the solution.” -
Newton’s Cradle – An AI Film By Jeff Synthesized
Narrative voice via Artlistai, News Reporter PlayAI, All other voices are V2V in Elevenlabs.
Powered by (in order of amount) ‘HailuoAI’, ‘KlingAI’ and of course some of our special source. Performance capture by ‘Runway’s Act-One’.
Edited and color graded in ‘DaVinci Resolve’. Composited with ‘After Effects’.
In this film, the ‘Newton’s Cradle’ isn’t just a symbolic object—it represents the fragile balance between control and freedom in a world where time itself is being manipulated. The oscillation of the cradle reflects the constant push and pull of power in this dystopian society. By the end of the film, we discover that this seemingly innocuous object holds the potential to disrupt the system, offering a glimmer of hope that time can be reset and balance restored. -
xinsir – controlnet-union-sdxl-1.0 examples
https://huggingface.co/xinsir/controlnet-union-sdxl-1.0
deblur
inpainting
outpainting
upscale
openpose
depthmap
canny
lineart
anime lineart
mlsd
scribble
hed
softedge
ted
segmentation
normals
openpose + canny
-
What is deepfake GAN (Generative Adversarial Network) technology?
https://www.techtarget.com/whatis/definition/deepfake
Deepfake technology is a type of artificial intelligence used to create convincing fake images, videos and audio recordings. The term describes both the technology and the resulting bogus content and is a portmanteau of deep learning and fake.
Deepfakes often transform existing source content where one person is swapped for another. They also create entirely original content where someone is represented doing or saying something they didn’t do or say.
Deepfakes aren’t edited or photoshopped videos or images. In fact, they’re created using specialized algorithms that blend existing and new footage. For example, subtle facial features of people in images are analyzed through machine learning (ML) to manipulate them within the context of other videos.
Deepfakes uses two algorithms — a generator and a discriminator — to create and refine fake content. The generator builds a training data set based on the desired output, creating the initial fake digital content, while the discriminator analyzes how realistic or fake the initial version of the content is. This process is repeated, enabling the generator to improve at creating realistic content and the discriminator to become more skilled at spotting flaws for the generator to correct.
The combination of the generator and discriminator algorithms creates a generative adversarial network.
A GAN uses deep learning to recognize patterns in real images and then uses those patterns to create the fakes.
When creating a deepfake photograph, a GAN system views photographs of the target from an array of angles to capture all the details and perspectives.
When creating a deepfake video, the GAN views the video from various angles and analyzes behavior, movement and speech patterns.
This information is then run through the discriminator multiple times to fine-tune the realism of the final image or video. -
Eddie Yoon – There’s a big misconception about AI creative
You’re being tricked into believing that AI can produce Hollywood-level videos…
We’re far from it.
Yes, we’ve made huge progress.
A video sample like this, created using Kling 1.6, is light-years ahead of what was possible a year ago. But there’s still a significant limitation: visual continuity beyond 5 seconds.
Right now, no AI model can maintain consistency beyond a few seconds. That’s why most AI-generated concepts you’re seeing on your feed rely on 2–5 second cuts – it’s all the tech can handle before things start to fall apart.
This isn’t necessarily a problem for creating movie trailers or spec ads. Trailers, for instance, are designed for quick, attention-grabbing rapid cuts, and AI excels at this style of visual storytelling.
But, making a popular, full-length movie with nothing but 5-second shots? That’s absurd.
There are very few exceptions to this rule in modern cinema (e.g., the Bourne franchise).
To bridge the gap between trailers and full-length cinema, AI creative needs to reach 2 key milestones:
– 5-12 sec average: ASL for slower, non-action scenes in contemporary films – think conversations, emotional moments, or establishing shots
– 30+ sec sequences: Longer, uninterrupted takes are essential for genres that require immersion – drama, romance, thrillers, or any scene that builds tension or atmosphere
Mastering longer cuts is crucial.
30-second continuous shots require a higher level of craftsmanship and visual consistency – you need that 20-30 seconds of breathing room to piece together a variety of scenes and create a compelling movie.
So, where does AI creative stand now?
AI is already transforming industries like auto, fashion, and CPG. These brands can use AI today because short, 2–5 second cuts work perfectly in their visual language. Consumers are accustomed to it, and it simply works. This psychological dynamic is unlikely to change anytime soon.
But for AI to produce true cinema (not just flashy concepts) it needs to extend its visual consistency. And every GenAI company is racing to get there.
The timeline?
Next year, expect breakthroughs in AI-generated content holding consistency for 10+ seconds. By then, full-length commercials, shows, and movies (in that order) will start to feel more crafted, immersive, and intentional, not just stitched together.
If you’re following AI’s impact on creativity, this is the development to watch. The companies that solve continuity will redefine what’s possible in film.
COLLECTIONS
| Featured AI
| Design And Composition
| Explore posts
POPULAR SEARCHES
unreal | pipeline | virtual production | free | learn | photoshop | 360 | macro | google | nvidia | resolution | open source | hdri | real-time | photography basics | nuke
FEATURED POSTS
-
Kling 1.6 and competitors – advanced tests and comparisons
-
Photography basics: Lumens vs Candelas (candle) vs Lux vs FootCandle vs Watts vs Irradiance vs Illuminance
-
Scene Referred vs Display Referred color workflows
-
Key/Fill ratios and scene composition using false colors
-
AI Data Laundering: How Academic and Nonprofit Researchers Shield Tech Companies from Accountability
-
NVidia – High-Fidelity 3D Mesh Generation at Scale with Meshtron
-
Rec-2020 – TVs new color gamut standard used by Dolby Vision?
-
STOP FCC – SAVE THE FREE NET
Social Links
DISCLAIMER – Links and images on this website may be protected by the respective owners’ copyright. All data submitted by users through this site shall be treated as freely available to share.
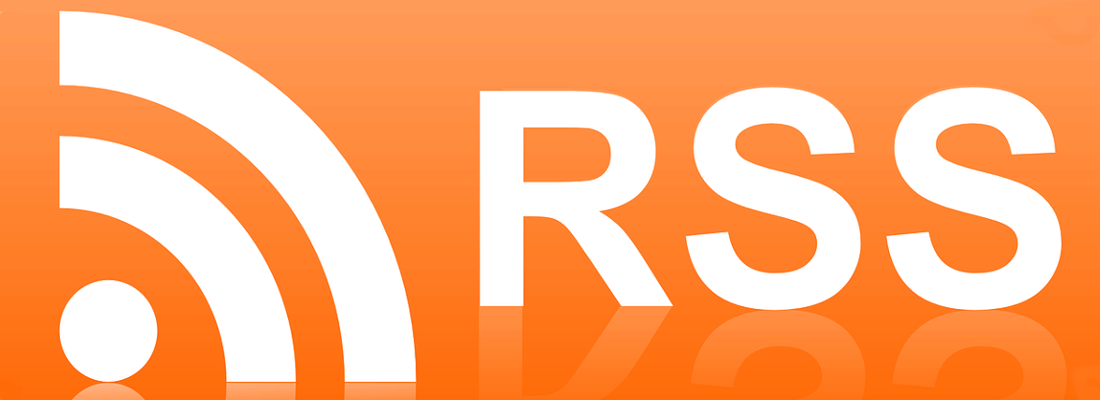