With this technique you can train even with 12gb VRAM.
https://github.com/kijai/ComfyUI-FluxTrainer
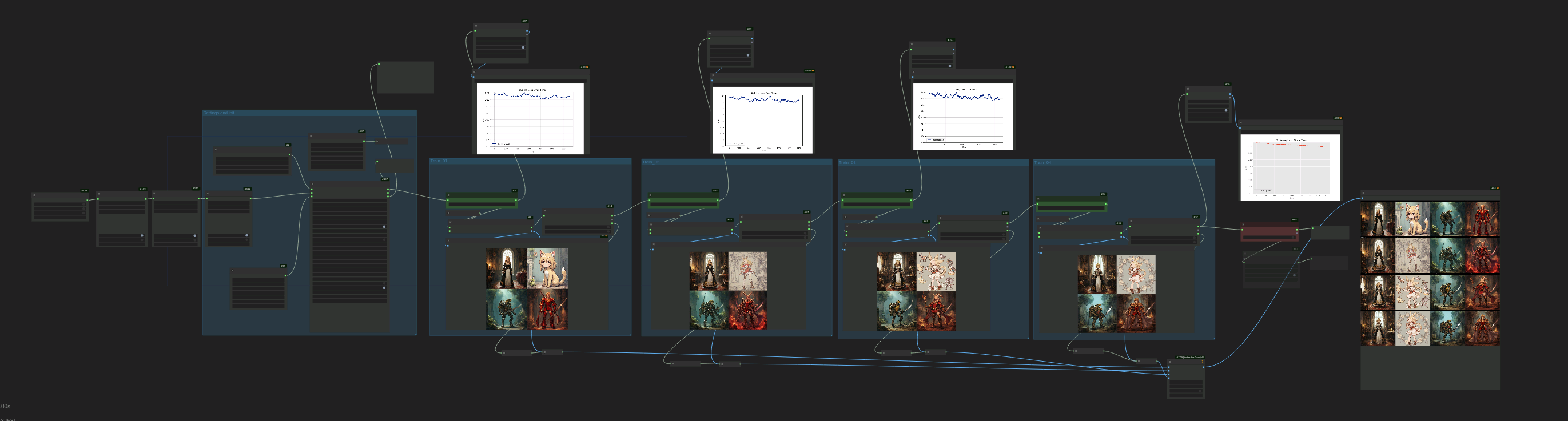
3Dprinting (176) A.I. (761) animation (340) blender (197) colour (229) commercials (49) composition (152) cool (360) design (636) Featured (69) hardware (308) IOS (109) jokes (134) lighting (282) modeling (131) music (186) photogrammetry (178) photography (751) production (1254) python (87) quotes (491) reference (310) software (1336) trailers (297) ves (538) VR (219)
A 2D Gaussian Splats technique for extracting cleaner 3D geometry from 3DGS
https://github.com/hbb1/2d-gaussian-splatting
https://surfsplatting.github.io/
https://colab.research.google.com/drive/1qoclD7HJ3-o0O1R8cvV3PxLhoDCMsH8W
3D Gaussian Splatting (3DGS) has recently revolutionized radiance field reconstruction, achieving high quality novel view synthesis and fast rendering speed without baking. However, 3DGS fails to accurately represent surfaces due to the multi-view inconsistent nature of 3D Gaussians. We present 2D Gaussian Splatting (2DGS), a novel approach to model and reconstruct geometrically accurate radiance fields from multi-view images. Our key idea is to collapse the 3D volume into a set of 2D oriented planar Gaussian disks. Unlike 3D Gaussians, 2D Gaussians provide view-consistent geometry while modeling surfaces intrinsically. To accurately recover thin surfaces and achieve stable optimization, we introduce a perspective-accurate 2D splatting process utilizing ray-splat intersection and rasterization. Additionally, we incorporate depth distortion and normal consistency terms to further enhance the quality of the reconstructions. We demonstrate that our differentiable renderer allows for noise-free and detailed geometry reconstruction while maintaining competitive appearance quality, fast training speed, and real-time rendering.
Kiosk streamlines resource management. With tailored filtering, customizable organization, and seamless integration into Maya, Houdini, Blender and Cinema4D. Maintain one library for them all!
https://fabianstrube.gumroad.com/l/kiosk-library
This repository is a collection of simple USD projects. Each project shows off a single feature or group of USD features.
https://github.com/ColinKennedy/USD-Cookbook
These stubs are designed to be used with a type checker like mypy
to provide static type checking of python code, as well as to provide analysis and completion in IDEs like PyCharm and VSCode (with Pylance).
The Holy Grail – https://github.com/ad-si/awesome-3d-printing
Built on top of a series of fine-tunes of Stable Diffusion, Flux, and Magnific-built models.
https://www.freepik.com/ai/image-generator
After Effects Gaussian Splatting Plugin:
https://aescripts.com/gaussian-splatting/?aff=60
Blender Gaussian Splatting Plugin: (kinda buggy)
https://github.com/ReshotAI/gaussian-splatting-blender-addon
PostShot
https://www.pixelsham.com/2024/04/03/jawset-postshot-run-gaussian-splatting-with-a-ui-on-your-pc/
https://www.anthropic.com/news/artifacts
Artifacts turn conversations with Claude into a more creative and collaborative experience.
HTMLrev by Devluc provides free HTML website templates built with vanilla CSS, Bootstrap, Tailwind, React, Vue, Nextjs, Nuxt, Svelte, Gatsby, Laravel, Astro and more.
Convert STL files to PNG heightmap images for use with 3D printing.
https://fenrus75.github.io/FenrusCNCtools/javascript/stl2png.html
https://medium.com/keentools/facetracker-for-blender-guide-4779590cb873
https://medium.com/keentools/facetracker-for-blender-guide-4779590cb873
https://keentools.io/products/facetracker-for-blender?utm_source=youtube&utm_medium=guide
It connects Nuke with the ComfyUI server, any plugin that comes out in ComfyUI can be used in nuke, rotos with sam, rescaling, image generation, inpaintins, normal generator, the nodes are IPAdapter, ControlNet, AnimateDiff, Flux etc.
https://github.com/vinavfx/nuke_comfyui
COLLECTIONS
| Featured AI
| Design And Composition
| Explore posts
POPULAR SEARCHES
unreal | pipeline | virtual production | free | learn | photoshop | 360 | macro | google | nvidia | resolution | open source | hdri | real-time | photography basics | nuke
FEATURED POSTS
Social Links
DISCLAIMER – Links and images on this website may be protected by the respective owners’ copyright. All data submitted by users through this site shall be treated as freely available to share.