-
Raw hours – If you want to get really good at something, forget about shortcuts. You simply have to inject a ton of raw hours.
Jonathan Stern Raw hours
On Twitter yesterday, @RJoads asked me how I got good at styling (CSS).
I replied: “Raw hours. I’m obsessed with how things look and feel—probably more than the median engineer. Mind you, this has not always been a positive. I’ve been wildly distracted for hours and hours on the smallest items, things that truly do not matter to the business. But that’s how I’ve gotten better.”
If you want to get really good at something, forget about shortcuts. You simply have to inject a ton of raw hours.
“Work smarter, not harder” is a common refrain these days—particularly in sophisticated circles. The thing is, for most people I think it’s bad advice. My experience learning to code has suggested you have to work hard before you know how to work smart. No substitute for raw hours.
It’s similar to what Brian Armstrong says: “If you’re pre-product/market fit, the best advice that I have from that period is: action produces information. Just keep doing stuff.”
People with limited experience are similar to startups pre-product/market fit. Of course you should aim to work smarter! The catch is that you have to work extremely hard in order to know HOW to work smart. You must first go down dozens of dead-end paths to know where the smarter paths lie.
The second catch is that in order to be able to inject a ton of raw hours in a natural, sustainable way, oftentimes you need to be OBSESSED. Otherwise – you’ll be banging your head against the wall year after year. Some people are so dogged they’re able to do it. But this is exceedingly rare and probably not worth aspiring to.
“It’s hard to do a really good job on anything you don’t think about in the shower.” – Paul Graham
Doing is normally distributed; Learning is log-normal
https://hiandrewquinn.github.io/til-site/posts/doing-is-normally-distributed-learning-is-log-normal
-
braindump.me – Building an AI game studio: what we’ve learned so far
https://braindump.me/blog-posts/building-an-ai-game-studio
Braindump is an attempt to imagine what game creation could be like in the brave new world of LLMs and generative AI to give you an entire AI game studio, complete with coders, artists, and so on, to help you create your dream game.
-
How we’ve failed the average worker and instead glorified CEOs
CEO total compensation has outpaced US median annual income by 16,638%, on average.
US median annual income increased by just 4% on average ($2,108/year).
CEO total compensation had an average annual increase of 7% ($676,153/year
Since 1974, CEO compensation has grown 940% while the average worker’s compensation has risen just 12%. Meanwhile, the purchasing power of the dollar over that same period has decreased an average of 3% a year from inflation. As a result, the average worker can afford significantly less goods and services today than they could 50 years ago, including housing, clothes and food. The average worker is losing big time.
And for those who would argue the high cost of social welfare, corporate welfare will cost taxpayers almost $400 billion this year alone, which is 25,000% higher than the $1.6 billion that will be spent on social welfare.
Sources
https://www.linkedin.com/posts/hannahawilliams_eattherich-activity-7198758131538046978-Vr2O
-
Godot Cheat Sheets
https://docs.godotengine.org/en/stable/tutorials/scripting/gdscript/gdscript_basics.html
https://www.canva.com/design/DAGBWXOIWXY/hW1uECYrkiyqs9rN0a-XIA/view?utm_content=DAGBWXOIWXY
https://www.reddit.com/r/godot/comments/18aid4u/unit_circle_in_godot_format_version_2_by_foxsinart/
Images in the post
<!–more–>
COLLECTIONS
| Featured AI
| Design And Composition
| Explore posts
POPULAR SEARCHES
unreal | pipeline | virtual production | free | learn | photoshop | 360 | macro | google | nvidia | resolution | open source | hdri | real-time | photography basics | nuke
FEATURED POSTS
Social Links
DISCLAIMER – Links and images on this website may be protected by the respective owners’ copyright. All data submitted by users through this site shall be treated as freely available to share.
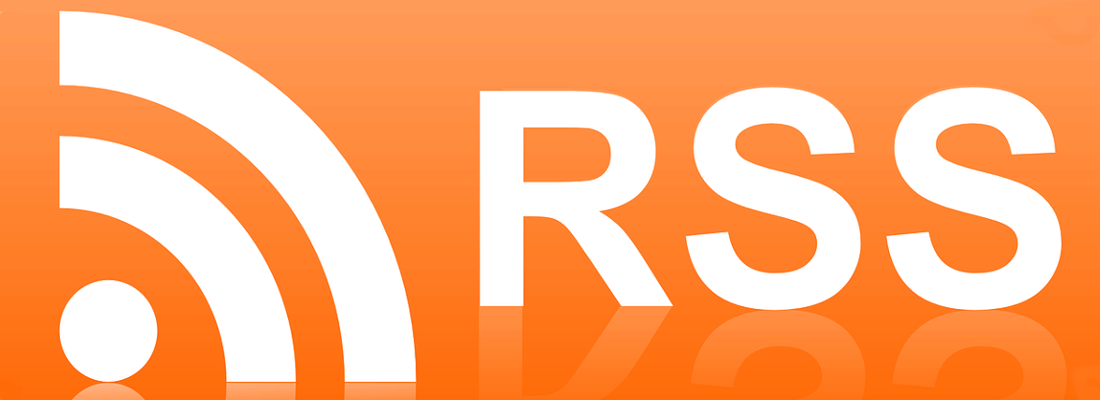