-
ComfyUI Tutorial Series Ep 25 – LTX Video โ Fast AI Video Generator Model
https://comfyanonymous.github.io/ComfyUI_examples/ltxv
LTX-Video 2B v0.9.1 Checkpoint model
https://huggingface.co/Lightricks/LTX-Video/tree/main
More details under the post
(more…) -
The AI-Copyright Trap document by Carys Craig
https://papers.ssrn.com/sol3/papers.cfm?abstract_id=4905118
“There are many good reasons to be concerned about the rise of generative AI(…). Unfortunately, there are also many good reasons to be concerned about copyrightโs growing prevalence in the policy discourse around AIโs regulation. Insisting that copyright protects an exclusive right to use materials for text and data mining practices (whether for informational analysis or machine learning to train generative AI models) is likely to do more harm than good. As many others have explained, imposing copyright constraints will certainly limit competition in the AI industry, creating cost-prohibitive barriers to quality data and ensuring that only the most powerful players have the means to build the best AI tools (provoking all of the usual monopoly concerns that accompany this kind of market reality but arguably on a greater scale than ever before). It will not, however, prevent the continued development and widespread use of generative AI.”
…
“(…) As Michal Shur-Ofry has explained, the technical traits of generative AI already mean that its outputs will tend towards the dominant, likely reflecting ‘a relatively narrow, mainstream view, prioritizing the popular and conventional over diverse contents and narratives.’ย Perhaps, then, if the political goal is to push for equality, participation, and representation in the AI age, criticsโ demands should focus not on exclusivity but inclusivity.ย If we want to encourage the development of ethical and responsible AI, maybe we should be asking what kind of material and training data must be included in the inputs and outputs of AI to advance that goal. Certainly, relying on copyright and the market to dictate what is in and what is out is unlikely to advance a public interest or equality-oriented agenda.”
…
“If copyright is not the solution, however, it might reasonably be asked: what is? The first step to answering that questionโto producing a purposively sound prescription and evidence-based prognosis, is to correctly diagnose the problem. If, as I have argued, the problem is not that AI models are being trained on copyright works without their ownersโ consent, then requiring copyright ownersโ consent and/or compensation for the use of their work in AI-training datasets is not the appropriate solution. (…)If the only real copyright problem is that the outputs of generative AI may be substantially similar to specific human-authored and copyright-protected works, then copyright law as we know it already provides the solution.” -
Newtonโs Cradle – An AI Film By Jeff Synthesized
Narrative voice via Artlistai, News Reporter PlayAI, All other voices are V2V in Elevenlabs.
Powered by (in order of amount) โHailuoAIโ, โKlingAIโ and of course some of our special source. Performance capture by โRunwayโs Act-Oneโ.
Edited and color graded in โDaVinci Resolveโ. Composited with โAfter Effectsโ.
In this film, the โNewtonโs Cradleโ isnโt just a symbolic objectโit represents the fragile balance between control and freedom in a world where time itself is being manipulated. The oscillation of the cradle reflects the constant push and pull of power in this dystopian society. By the end of the film, we discover that this seemingly innocuous object holds the potential to disrupt the system, offering a glimmer of hope that time can be reset and balance restored. -
xinsir – controlnet-union-sdxl-1.0 examples
https://huggingface.co/xinsir/controlnet-union-sdxl-1.0
deblur
inpainting
outpainting
upscale
openpose
depthmap
canny
lineart
anime lineart
mlsd
scribble
hed
softedge
ted
segmentation
normals
openpose + canny
-
What is deepfake GAN (Generative Adversarial Network) technology?
https://www.techtarget.com/whatis/definition/deepfake
Deepfake technology is a type of artificial intelligence used to create convincing fake images, videos and audio recordings. The term describes both the technology and the resulting bogus content and is a portmanteau of deep learning and fake.
Deepfakes often transform existing source content where one person is swapped for another. They also create entirely original content where someone is represented doing or saying something they didn’t do or say.
Deepfakes aren’t edited or photoshopped videos or images. In fact, they’re created using specialized algorithms that blend existing and new footage. For example, subtle facial features of people in images are analyzed through machine learning (ML) to manipulate them within the context of other videos.
Deepfakes uses two algorithms — a generator and a discriminator — to create and refine fake content. The generator builds a training data set based on the desired output, creating the initial fake digital content, while the discriminator analyzes how realistic or fake the initial version of the content is. This process is repeated, enabling the generator to improve at creating realistic content and the discriminator to become more skilled at spotting flaws for the generator to correct.
The combination of the generator and discriminator algorithms creates a generative adversarial network.
A GAN uses deep learning to recognize patterns in real images and then uses those patterns to create the fakes.
When creating a deepfake photograph, a GAN system views photographs of the target from an array of angles to capture all the details and perspectives.
When creating a deepfake video, the GAN views the video from various angles and analyzes behavior, movement and speech patterns.
This information is then run through the discriminator multiple times to fine-tune the realism of the final image or video. -
The History, Evolution and Rise of AI
https://medium.com/@lmpo/a-brief-history-of-ai-with-deep-learning-26f7948bc87b
๐น 1943: ๐ ๐ฐ๐๐๐น๐น๐ผ๐ฐ๐ต & ๐ฃ๐ถ๐๐๐ create the first artificial neuron.
๐น 1950: ๐๐น๐ฎ๐ป ๐ง๐๐ฟ๐ถ๐ป๐ด introduces the Turing Test, forever changing the way we view intelligence.
๐น 1956: ๐๐ผ๐ต๐ป ๐ ๐ฐ๐๐ฎ๐ฟ๐๐ต๐ coins the term โArtificial Intelligence,โ marking the official birth of the field.
๐น 1957: ๐๐ฟ๐ฎ๐ป๐ธ ๐ฅ๐ผ๐๐ฒ๐ป๐ฏ๐น๐ฎ๐๐ invents the Perceptron, one of the first neural networks.
๐น 1959: ๐๐ฒ๐ฟ๐ป๐ฎ๐ฟ๐ฑ ๐ช๐ถ๐ฑ๐ฟ๐ผ๐ and ๐ง๐ฒ๐ฑ ๐๐ผ๐ณ๐ณ create ADALINE, a model that would shape neural networks.
๐น 1969: ๐ ๐ถ๐ป๐๐ธ๐ & ๐ฃ๐ฎ๐ฝ๐ฒ๐ฟ๐ solve the XOR problem, but also mark the beginning of the “first AI winter.”
๐น 1980: ๐๐๐ป๐ถ๐ต๐ถ๐ธ๐ผ ๐๐๐ธ๐๐๐ต๐ถ๐บ๐ฎ introduces Neocognitron, laying the groundwork for deep learning.
๐น 1986: ๐๐ฒ๐ผ๐ณ๐ณ๐ฟ๐ฒ๐ ๐๐ถ๐ป๐๐ผ๐ป and ๐๐ฎ๐๐ถ๐ฑ ๐ฅ๐๐บ๐ฒ๐น๐ต๐ฎ๐ฟ๐ introduce backpropagation, making neural networks viable again.
๐น 1989: ๐๐๐ฑ๐ฒ๐ฎ ๐ฃ๐ฒ๐ฎ๐ฟ๐น advances UAT (Understanding and Reasoning), building a foundation for AI’s logical abilities.
๐น 1995: ๐ฉ๐น๐ฎ๐ฑ๐ถ๐บ๐ถ๐ฟ ๐ฉ๐ฎ๐ฝ๐ป๐ถ๐ธ and ๐๐ผ๐ฟ๐ถ๐ป๐ป๐ฎ ๐๐ผ๐ฟ๐๐ฒ๐ develop Support Vector Machines (SVMs), a breakthrough in machine learning.
๐น 1998: ๐ฌ๐ฎ๐ป๐ป ๐๐ฒ๐๐๐ป popularizes Convolutional Neural Networks (CNNs), revolutionizing image recognition.
๐น 2006: ๐๐ฒ๐ผ๐ณ๐ณ๐ฟ๐ฒ๐ ๐๐ถ๐ป๐๐ผ๐ป and ๐ฅ๐๐๐น๐ฎ๐ป ๐ฆ๐ฎ๐น๐ฎ๐ธ๐ต๐๐๐ฑ๐ถ๐ป๐ผ๐ introduce deep belief networks, reigniting interest in deep learning.
๐น 2012: ๐๐น๐ฒ๐ ๐๐ฟ๐ถ๐๐ต๐ฒ๐๐๐ธ๐ and ๐๐ฒ๐ผ๐ณ๐ณ๐ฟ๐ฒ๐ ๐๐ถ๐ป๐๐ผ๐ป launch AlexNet, sparking the modern AI revolution in deep learning.
๐น 2014: ๐๐ฎ๐ป ๐๐ผ๐ผ๐ฑ๐ณ๐ฒ๐น๐น๐ผ๐ introduces Generative Adversarial Networks (GANs), opening new doors for AI creativity.
๐น 2017: ๐๐๐ต๐ถ๐๐ต ๐ฉ๐ฎ๐๐๐ฎ๐ป๐ถ and team introduce Transformers, redefining natural language processing (NLP).
๐น 2020: OpenAI unveils GPT-3, setting a new standard for language models and AIโs capabilities.
๐น 2022: OpenAI releases ChatGPT, democratizing conversational AI and bringing it to the masses. -
Eddie Yoon – There’s a big misconception about AI creative
You’re being tricked into believing that AI can produce Hollywood-level videos…
Weโre far from it.
Yes, weโve made huge progress.
A video sample like this, created using Kling 1.6, is light-years ahead of what was possible a year ago. But thereโs still a significant limitation: visual continuity beyond 5 seconds.
Right now, no AI model can maintain consistency beyond a few seconds. Thatโs why most AI-generated concepts youโre seeing on your feed rely on 2โ5 second cuts – itโs all the tech can handle before things start to fall apart.
This isnโt necessarily a problem for creating movie trailers or spec ads. Trailers, for instance, are designed for quick, attention-grabbing rapid cuts, and AI excels at this style of visual storytelling.
But, making a popular, full-length movie with nothing but 5-second shots? Thatโs absurd.
There are very few exceptions to this rule in modern cinema (e.g., the Bourne franchise).
To bridge the gap between trailers and full-length cinema, AI creative needs to reach 2 key milestones:
– 5-12 sec average: ASL for slower, non-action scenes in contemporary films – think conversations, emotional moments, or establishing shots
– 30+ sec sequences: Longer, uninterrupted takes are essential for genres that require immersion – drama, romance, thrillers, or any scene that builds tension or atmosphere
Mastering longer cuts is crucial.
30-second continuous shots require a higher level of craftsmanship and visual consistency – you need that 20-30 seconds of breathing room to piece together a variety of scenes and create a compelling movie.
So, where does AI creative stand now?
AI is already transforming industries like auto, fashion, and CPG. These brands can use AI today because short, 2โ5 second cuts work perfectly in their visual language. Consumers are accustomed to it, and it simply works. This psychological dynamic is unlikely to change anytime soon.
But for AI to produce true cinema (not just flashy concepts) it needs to extend its visual consistency. And every GenAI company is racing to get there.
The timeline?
Next year, expect breakthroughs in AI-generated content holding consistency for 10+ seconds. By then, full-length commercials, shows, and movies (in that order) will start to feel more crafted, immersive, and intentional, not just stitched together.
If youโre following AIโs impact on creativity, this is the development to watch. The companies that solve continuity will redefine whatโs possible in film.
-
Andreas Horn – Want cutting edge AI?
๐ง๐ต๐ฒ ๐ฏ๐๐ถ๐น๐ฑ๐ถ๐ป๐ด ๐ฏ๐น๐ผ๐ฐ๐ธ๐ ๐ผ๐ณ ๐๐ ๐ฎ๐ป๐ฑ ๐ฒ๐๐๐ฒ๐ป๐๐ถ๐ฎ๐น ๐ฝ๐ฟ๐ผ๐ฐ๐ฒ๐๐๐ฒ๐:
– Collect: Data from sensors, logs, and user input.
– Move/Store: Build infrastructure, pipelines, and reliable data flow.
– Explore/Transform: Clean, prep, and detect anomalies to make the data usable.
– Aggregate/Label: Add analytics, metrics, and labels to create training data.
– Learn/Optimize: Experiment, test, and train AI models.๐ง๐ต๐ฒ ๐น๐ฎ๐๐ฒ๐ฟ๐ ๐ผ๐ณ ๐ฑ๐ฎ๐๐ฎ ๐ฎ๐ป๐ฑ ๐ต๐ผ๐ ๐๐ต๐ฒ๐ ๐ฏ๐ฒ๐ฐ๐ผ๐บ๐ฒ ๐ถ๐ป๐๐ฒ๐น๐น๐ถ๐ด๐ฒ๐ป๐:
– Instrumentation and logging: Sensors, logs, and external data capture the raw inputs.
– Data flow and storage: Pipelines and infrastructure ensure smooth movement and reliable storage.
– Exploration and transformation: Data is cleaned, prepped, and anomalies are detected.
– Aggregation and labeling: Analytics, metrics, and labels create structured, usable datasets.
– Experimenting/AI/ML: Models are trained and optimized using the prepared data.
– AI insights and actions: Advanced AI generates predictions, insights, and decisions at the top.๐ช๐ต๐ผ ๐บ๐ฎ๐ธ๐ฒ๐ ๐ถ๐ ๐ต๐ฎ๐ฝ๐ฝ๐ฒ๐ป ๐ฎ๐ป๐ฑ ๐ธ๐ฒ๐ ๐ฟ๐ผ๐น๐ฒ๐:
– Data Infrastructure Engineers: Build the foundation โ collect, move, and store data.
– Data Engineers: Prep and transform the data into usable formats.
– Data Analysts & Scientists: Aggregate, label, and generate insights.
– Machine Learning Engineers: Optimize and deploy AI models.๐ง๐ต๐ฒ ๐บ๐ฎ๐ด๐ถ๐ฐ ๐ผ๐ณ ๐๐ ๐ถ๐ ๐ถ๐ป ๐ต๐ผ๐ ๐๐ต๐ฒ๐๐ฒ ๐น๐ฎ๐๐ฒ๐ฟ๐ ๐ฎ๐ป๐ฑ ๐ฟ๐ผ๐น๐ฒ๐ ๐๐ผ๐ฟ๐ธ ๐๐ผ๐ด๐ฒ๐๐ต๐ฒ๐ฟ. ๐ง๐ต๐ฒ ๐๐๐ฟ๐ผ๐ป๐ด๐ฒ๐ฟ ๐๐ผ๐๐ฟ ๐ณ๐ผ๐๐ป๐ฑ๐ฎ๐๐ถ๐ผ๐ป, ๐๐ต๐ฒ ๐๐บ๐ฎ๐ฟ๐๐ฒ๐ฟ ๐๐ผ๐๐ฟ ๐๐.
COLLECTIONS
| Featured AI
| Design And Composition
| Explore posts
POPULAR SEARCHES
unreal | pipeline | virtual production | free | learn | photoshop | 360 | macro | google | nvidia | resolution | open source | hdri | real-time | photography basics | nuke
FEATURED POSTS
Social Links
DISCLAIMER – Links and images on this website may be protected by the respective ownersโ copyright. All data submitted by users through this site shall be treated as freely available to share.
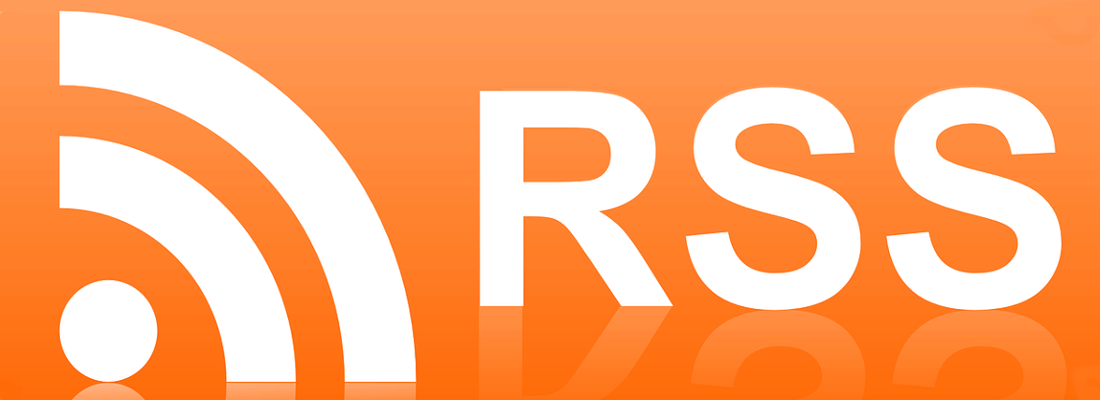